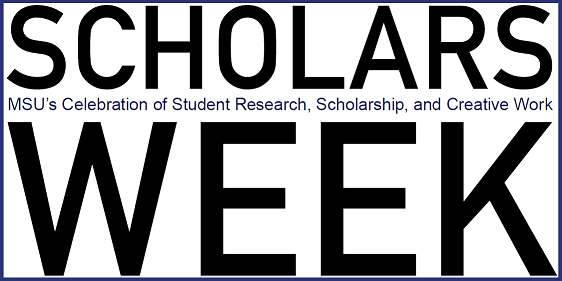
Sigma Xi Poster Competition
Turbidity Mapping of the Ohio River using In Situ and Satellite Imagery
Academic Level at Time of Presentation
Junior
Major
Earth & Environmental Science/ Geography & GIS
List all Project Mentors & Advisor(s)
Dr. Haluk Cetin
Presentation Format
Poster Presentation
Abstract/Description
Turbidity mapping of the Ohio River using in situ and satellite imagery
As urban populations and agricultural lands grow in size, water quality has become one of the foremost environmental issues. The quality of our waterways has tremendous impact on recreation, aquatic life, drinking water, and much more. In this study, turbidity was evaluated by using remotely sensed imagery and USGS station gage turbidity data for the year of 2020 along the Ohio River. The USGS turbidity data were then compared to the individual band values of Landsat 8 imagery at each station along the Ohio River. These data were put into a linear regression model where it was found that band 4, the red band, of the Landsat 8 data had a moderately high correlation with turbidity at an R2 value of 0.71. Surface reflectance images were reclassified to create a “water-only” image. Using model maker in ERDAS Imagine, the water only image was put into the linear regression equation to map the turbidity of the Ohio River.
Spring Scholars Week 2022 Event
Sigma Xi Poster Competition
Turbidity Mapping of the Ohio River using In Situ and Satellite Imagery
Turbidity mapping of the Ohio River using in situ and satellite imagery
As urban populations and agricultural lands grow in size, water quality has become one of the foremost environmental issues. The quality of our waterways has tremendous impact on recreation, aquatic life, drinking water, and much more. In this study, turbidity was evaluated by using remotely sensed imagery and USGS station gage turbidity data for the year of 2020 along the Ohio River. The USGS turbidity data were then compared to the individual band values of Landsat 8 imagery at each station along the Ohio River. These data were put into a linear regression model where it was found that band 4, the red band, of the Landsat 8 data had a moderately high correlation with turbidity at an R2 value of 0.71. Surface reflectance images were reclassified to create a “water-only” image. Using model maker in ERDAS Imagine, the water only image was put into the linear regression equation to map the turbidity of the Ohio River.